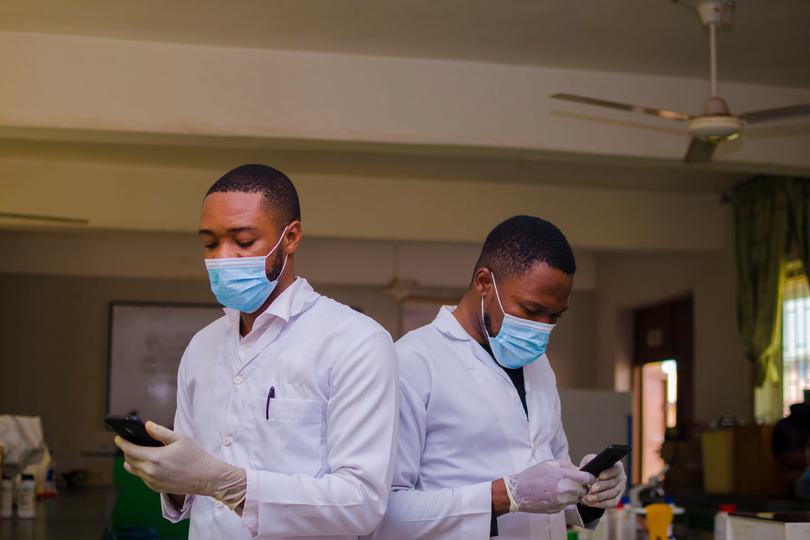
The Oxford Martin Programme on Global Epilepsy has worked tirelessly to improve diagnoses in the developing world. On International Epilepsy Day, we explore what potential there is for deploying affordable digital diagnostic tools in such countries and what challenges lie ahead
Epilepsy is a common neurological condition that disproportionately affects people from disadvantaged socio-economic groups. According to the WHO, around 50 million people have epilepsy worldwide, with approximately 80% in low- and middle-income countries (LMICs). Diagnosing epilepsy requires (often expensive) training, skilled personnel, time and additional resources often not available in LMICs such as access to specialised equipment like electroencephalograms (EEGs).
The WHO also says that while an estimated 70% of people with epilepsy could live seizure-free with anti-seizure medications, over 75% of those living with epilepsy in LMICs cannot obtain a timely and appropriate diagnosis or subsequently any treatment. In such settings, affordable and accessible diagnostic tools that require less expertise, experience, or specialist training could empower primary healthcare workers to triage and prioritise people who may have epilepsy.
There is a clear need for comprehensive, high-quality data from a wide variety of settings and regions around the world.
Clinical machine learning (ML) models offer a practical solution to aid epilepsy diagnosis in low-resource settings, especially with mobile phone ownership on the rise. Such models have demonstrated promising outcomes for epilepsy diagnosis and treatment. As a result, it might be tempting to see them as an attractive one-size-fits-all solution – in particular as a potential solution to the diagnosis gap. However, given the enormous impact such models could stand to have, it’s crucial to ensure they are relevant, robust and appropriate, especially as they may be the only recourse for many users.
Our research has found that ML models developed on data from one region are not reliable for use elsewhere without prior validation. Previously, our group developed a predictive model to support epilepsy diagnosis in LMICs. In an upcoming study (available as a preprint), we assessed the performance of such diagnostic models in new settings so that we could evaluate how much we needed to adapt them across different locations. We are currently investigating the suitability of diagnostic models for deployment in regions that did not contribute data when the models were trained.
A prototype of a mobile electroencephalogram (EEG), which measures electrical activity in the brain and helps with epilepsy diagnoses
The measure of how well a new model works in new settings is known as ‘generalisability’. Failure of a model to generalise sufficiently to a new setting can be due to, for example, differences across the regions in how symptoms present or are reported by individuals. This is particularly applicable in epilepsy, where clinical diagnosis is primarily based on self-reported history and can be nuanced.
There is also the risk of the model ‘overfitting’, which means it has learned to make predictions based on biases in the dataset rather than developing a robust way to distinguish between the right diagnostic criteria. In the case of diagnostic tools, this can have significant consequences for the population in the location where the model is deployed, resulting in missed cases, over-diagnosis, wasted resources and potentially mistrust of the technology. These factors and the differences in clinical phenomenology and individual self-reporting highlight the need to validate models carefully before they are applied in new contexts.
Our findings suggest that site-specific validation is essential before a predictive model is deployed in practice. While complete re-training of all model parameters on local data would be ideal and result in optimal performance, this is not always practical. Studies have shown that it may suffice to adjust only a few parameters to successfully adapt a model to new settings.
Over 75% of those living with epilepsy in LMICs cannot obtain a timely and appropriate diagnosis or subsequently any treatment
As performance is continually assessed, we propose that iteratively updating the model throughout deployment can help fine-tune it further, with new improvements building upon previous findings. Such a model would generally require a threshold to determine positive cases, although, as shown, optimal thresholds vary between sites. The results suggest that simply validating a model’s threshold may also effectively update its performance in a new setting. In this study, we observed a mean change in accuracy of 10% following a threshold adjustment.
Finally, there is a clear need for comprehensive, high-quality data from a wide variety of settings and regions around the world. Large-scale datasets of this type would help mitigate the fear of overfitting or inappropriate over-specialisation to a particular setting, enabling models to be robust, reliable and accurate to the populations they aim to serve.
In harnessing the power of machine learning for epilepsy diagnosis in LMICs, we are working towards a future where early detection and accessible care become a reality for all — turning technological potential into lifesaving impact.
This opinion piece reflects the views of the author, and does not necessarily reflect the position of the Oxford Martin School or the University of Oxford. Any errors or omissions are those of the author.